PROJECT
The DAIS project will research and deliver Distributed Artificial Intelligent Systems. It will not research new algorithms, as such, but solves the problems of running existing algorithms on these vastly distributed edge devices that are designed based on these three European principles. The research and innovation activities are organized around eight different supply chains. Five of these focus on delivering the hard and software that is needed to run industrial grade AI on different type of networking topologies. Three of the supply chains demonstrate how known AI challenges, from different functional areas, are met by this pan European effort.
The DAIS project consists of 47 parties from 11 different countries. The DAIS project fosters cooperation between large and leading industrial players from different domains, a number of highly innovative SMEs, and cutting-edge research organisations and universities from all over Europa. Each of the supply chains, in which these parties collaborate, delivers its findings for broader dissemination in a special work package to directly influence industrial standards. The DAIS project aims to tilt the global competitive scale, in this key ECS domain, in Europe’s favour. The result will be that Europe’s industry will stay at the global forefront with a majority of its components proudly showing Made in Europe logos.
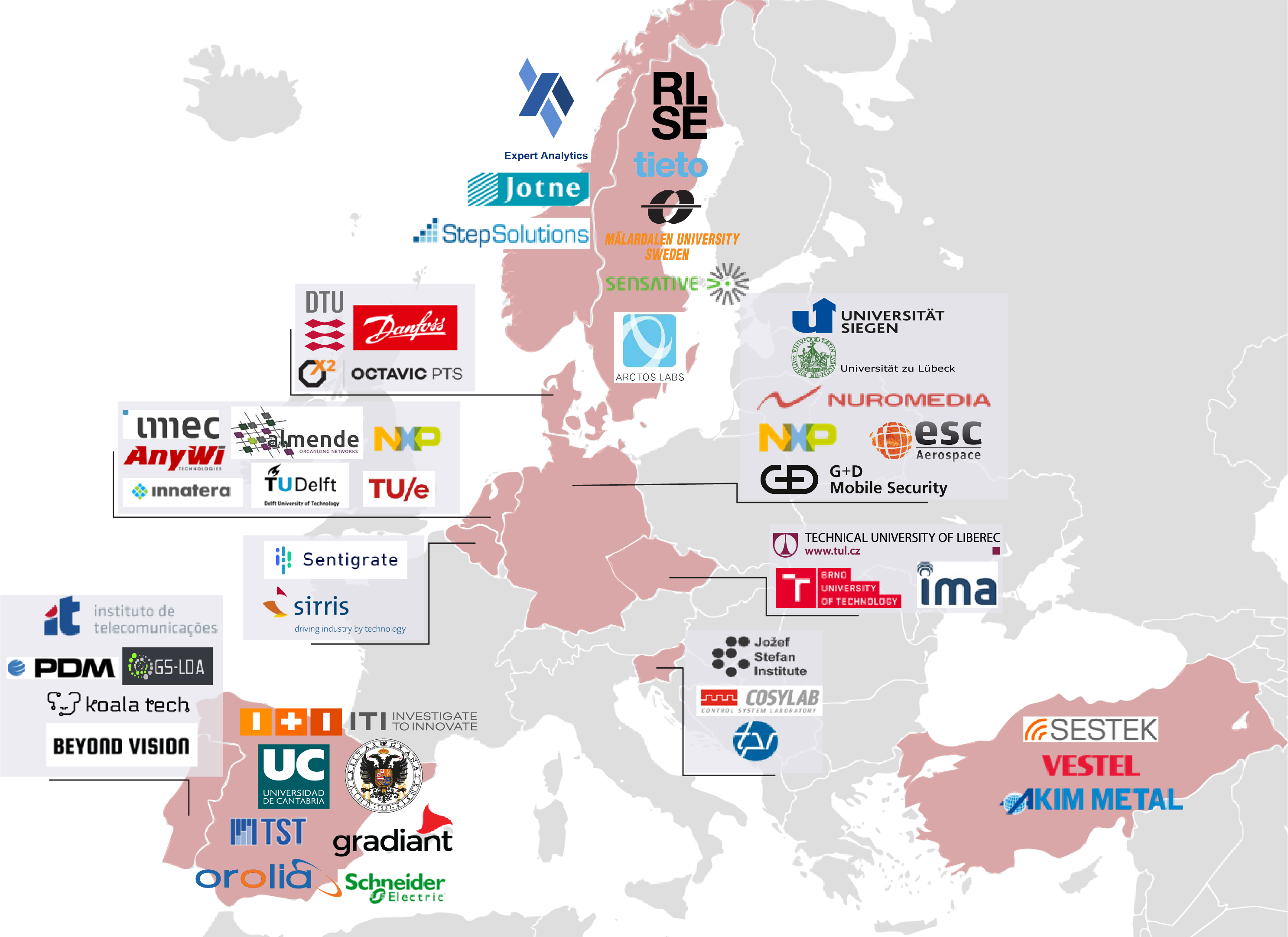
In recent years, technological developments in consumer electronics and industrial applications have been advancing rapidly. More and smaller, networked devices are able to collect and process data anywhere. This Internet of Things (IoT) is a revolutionary change for many sectors like building, automotive, digital industry, energy, etc. As a result, the amount of data being generated at the Edge level has and will increase dramatically, resulting in higher network bandwidth requirements. In the meantime, with the emergence of novel applications, lower latency of the network is required. The new paradigm of edge computing (EC) provides new solutions by bringing resources closer to the user, keeps sensitive & private data on device, and provides low latency, energy efficiency, and scalability compared to cloud services while reducing the network bandwidth. This in addition brings cost savings. EC guarantees quality of service when dealing with a massive amount of data for cloud computing1. Cisco Global Cloud Index2 estimates that there will be 10 times more useful data being created (85 ZB) than being stored or used (7.2 ZB) by 2021, and EC is a potential technology to help bridge this gap.
At the same time, Artificial Intelligence (AI) applications based on machine learning (especially deep learning algorithms) are being fuelled by advances in models, processing power, and big data. The developments of AI applications mostly require processing of data in centralized cloud locations and hence cannot be used for applications where milliseconds matter or for safety-critical applications. For example, as the sensors and cameras mounted on an autonomous vehicle generate about a gigabyte of data per second3, it is difficult, if not impossible to upload this data and get instructions from the cloud in real-time. Similarly, for face recognition and speech translation applications, they have high temporal requirements for processing either online or offline. Moreover, edge computing offers security benefits due to wider data distribution at the edge level. Reducing the distance data has to travel for processing means decreasing the opportunities for trackers and hackers to intercept it during transmission and preserves its privacy. With more data remaining at the edges of the network, central servers are also less likely to become targets for cyberattacks.
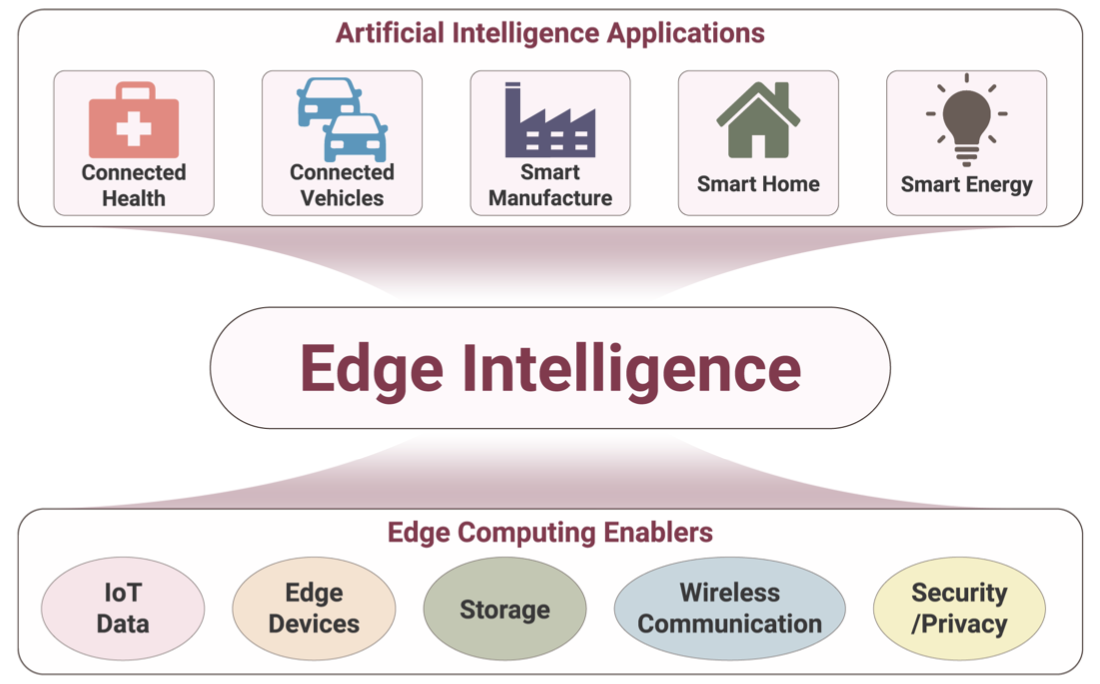