General Overview and Concept
In recent years, technological developments in consumer electronics and industrial applications have been advancing rapidly. More and more, small, networked devices are able to collect and process data anywhere. This Internet of Things (IoT) is a revolutionary change for many sectors like building, automotive, digital industry, energy, etc. As a result, the amount of data being generated at the Edge level has and will increase dramatically, resulting in higher network bandwidth requirements. In the meantime, with the emergence of novel applications, lower latency of the network is required. The new paradigm of edge computing (EC) provides new solutions by bringing resources closer to the user, keeps sensitive & private data on device, and provides low latency, energy efficiency, and scalability compared to cloud services while reducing the network bandwidth.
At the same time, there is an increasing need to use Artificial Intelligence (AI) at the edge. Today, AI applications based on machine learning (especially deep learning algorithms) are being fuelled by advances in models, processing power, and big data. The developments of AI applications mostly require processing of data in centralized cloud locations and hence cannot be used for applications where milliseconds matter in safety-critical applications, such as in autonomous vehicles, for example. Similarly, for face recognition and speech translation applications, there is high temporal requirements for processing either online or offline. In addition to speed, edge computing offers security benefits due to wider data distribution at the edge level. Reducing the distance data has to travel for processing means decreasing the opportunities for trackers and hackers to intercept it during transmission and preserves its privacy. With more data remaining at the edges of the network, central servers are also less likely to become targets for cyberattacks.
Migrating the AI functions from the cloud to the edge is highly regarded by industry and academy. In the Edge Intelligence (EI) scenario, advanced AI and machine learning algorithms are optimized to run on the edge capable of dealing features like video frames, natural speech information, time-series data and unstructured data generated by cameras, microphones, and other sensors without uploading data to the cloud and waiting for the response. However, the edge capability depends on the application and several practical factors, such as memory availability, performance needs, cost, and energy consumption. This will determine how much inferencing and analysis can be done at the edge leading to the common solution of intelligence being distributed between the cloud and edge device.
Overall Objectives of DAIS – Competitiveness for strong European Industry
DAIS has ambitious objective to develop Intelligent and Secure Edge solutions for industrial applications for European industry throughout the whole Supply Chain. More precisely, we do so by:
- Providing intelligent processing of data and communication locally at the edge to enable real-time and safety-critical industrial applications.
- Developing industrial-grade secure, safe and reliable solutions that can cope with cyberattacks and difficult network conditions.
- Providing AI techniques on the edge that match with diverse computing powers contrary to relatively consistent computing power on the cloud. As different AI algorithms have different computing power requirements, it is a big challenge to match an existing algorithm with the certain edge platform.
- Distribute and divide the complex AI operations between the cloud and edge, with edge undertaking early intelligent data processing reducing the bandwidth of data being transmitted to cloud; and building the hardware and software infrastructure to provide for this in Europe.
- Providing data sharing and collaborating solutions on the edge to handle the temporal-spatial diversity of edge data.
- Developing solutions for IoT, i.e. mostly wireless devices with energy- and processing- constraints, in heterogeneous and also hostile/harsh environments.
- Providing re-usable solutions across industrial domains.
- Methodological approach with the Integral Supply Chain, from academic, to system designers and integrators, to component providers, applications and services developers & providers and end users
Overall DAIS concept
The partners within DAIS conduct advanced research and development targeting to solutions towards the objectives as listed above. In order to achieve and validate the stated impact, the DAIS consortium has defined so-called supply chains (SC). A supply chain is the logical/virtual combination of partner activities fitting together within a specific topic and leading to a combined result, such as a demonstrator for the technologies developed. Each supply chain addresses a specific DAIS objective, and its results are used as input to other supply chains and project activities.
DAIS defines two types of supply chains:
- The enabling technology supply chains (1-5) develop the fundamental core SW and HW technology elements (such as components, systems and architectures) required by other supply chains (6-8) and for achieving the ambitious goals of DAIS.
- The application supply chains (6-8) will employ and validate the results achieved in the enabling technology supply chains. The advancements achieved during the DAIS project will be showcased by dedicated demonstrators of the supply chains 6-8.
Every effort and activity of a specific partner in a supply chain is mapped into the work packages, starting with requirements and ending with validation and test. In addition, each supply chain clearly addresses a specific DAIS objective. This approach forms a clearly arranged matrix structure, which is depicted in Figure 2. This ensures a clear understanding of responsibilities for project results and the achievement of project objectives, by assigning a lead for each supply chain and well-defined interfaces towards the WPs as well as to parallel supply chains. Consequently, this matrix structure supports the effective and efficient collaboration between the partners in a focused manner.
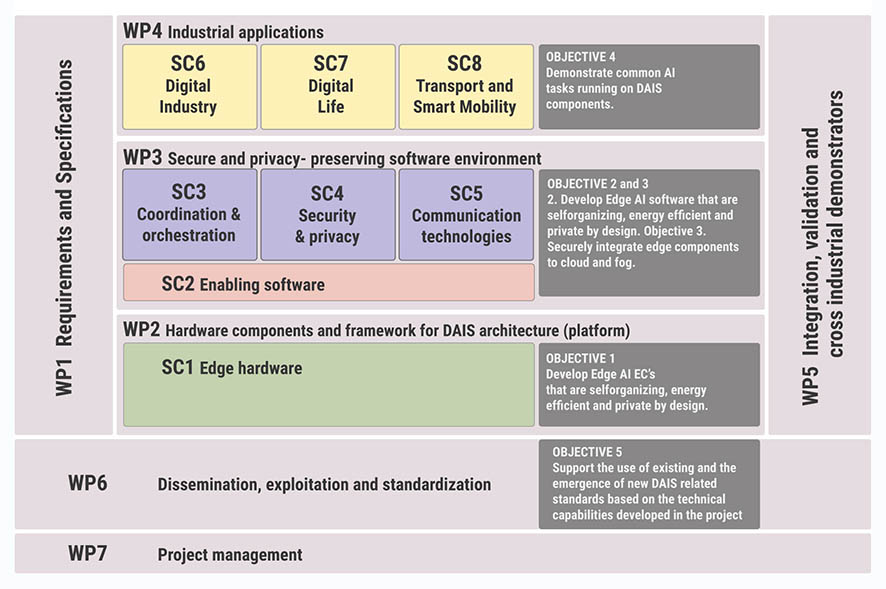