Coordination and orchestration of distributed AI
Current central cloud based IoT architectures generating massive amounts of data as result of billions of connected devices are not a viable solution for targeted distributed analytics. Therefore, the goal of coordination and orchestration is to enable a distributed AI solution between resource-constrained devices, fog and cloud (HW & SW), using security and communications capabilities, supporting dynamic data acquisition adapted to the specific context of Digital Industry, Digital life and Smart Mobility as well as more wide contexts that will be demonstrated and validated in DAIS cross-domain demonstrations. This will result in context-driven AI computation and results leveraging relevant historical and real-time data with relevant information based on alarms and notifications.
The distribution of compute-intensive tasks to local resources as far as possible will increase the scalability and lead to considerable improvements in response times and reduced downtime for real-time critical applications while the use of cloud computing enables the inclusion of cloud-based data sources in the AI models.
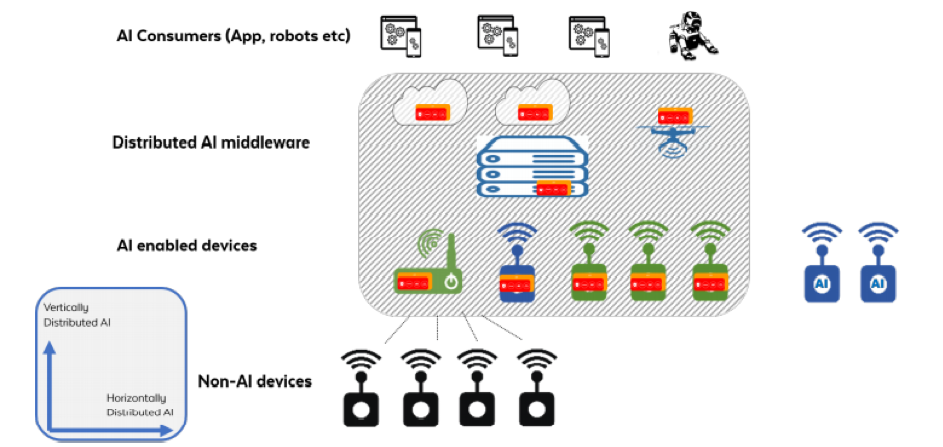
The goal of the work is to provide an open DAIS AI Framework so that AI computation can be distributed to edge, fog and cloud nodes in a federated manner by employing AI/ML based algorithms and mechanisms for energy- and resource-efficient planning, deployment and run-time adaptation of AI-based computing applications. The envisioned approach focuses on scaling of AI-based edge, fog & cloud computing applications, through their orchestration and distribution among a 2-dimensionally distributed infrastructure. Distributed and composable ML models and techniques that scale horizontally and/or vertically are the basis for the applications to guarantee high-quality decision making. This will empower edge computing to provide real-time processing and analytics capabilities near the point of use and source of critical real-time data and will significantly reduce the need for the expensive and relatively slow connection to the cloud as a bottleneck to analysis, especially when it comes to large volumes of data for AI applications. At the same time, the vertical distribution enables inclusion of data from multiple remote sources, including both internal and external data sources. Data collection, machine learning and inference tasks of AI applications can be distributed in a federated architecture. Such a solution is more robust and resilient as tasks can migrate in case of component failures.
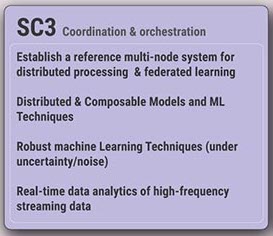
Objectives
1.) Reduce development, deployment and operation costs compared to existing solutions due the development of a DAIS AI Framework and utilization of otherwise unused computational resources.
2.) Increase IoT adoption through enhanced data control & privacy – increase privacy and security of cloud, fog and edge computing applications.
3.) Achieve high scalability thanks to the amount of pooled resources as well as distributed and composable ML models.
4.) Achieve means to share data and AI results between different networks, verticals and actors in a smart society.
5.) Utilize the benefits of both cloud-based AI and edge-based AI computing.
6.) Performance
- Low latency
- High availability – reduce downtime when operation under noise compared to a centralized approach.
- Reduce bandwidth requirements.
- Achieve the same performance and accuracy with the AI-driven distributed positioning engine as with the cloud-based solution.
- An improved performance of the local energy community when both optimization and engagement algorithms work in tandem rather than in isolation.
7.) Enable secure and privacy-aware data exchange between edge devices at public and private spaces.
8.) A base neural network trained for a specific problem type which may be transferred to similar problems and environments.
9.) Best practice method for setting up a neural network for the given problem type.
Results:
Techniques, components, and tools in order to establish and demonstrate a 2- dimensionally distributed AI.
Partners:
SENS (Leader), SIRRIS, SENT, CENT, Vestel, PDM, UCAN, Jotne, XAL, Arctos, RISE, ALM, TUE, NXPGE, Tieto, MDH, Gradiant, ITI, Sestek